In this PySpark quick start, let’s cover Apache Spark with Python fundamentals to get you started and feeling comfortable about using PySpark.
The intention is for readers to understand basic PySpark concepts through examples. Later posts will deeper dive into Apache Spark fundamentals and example use cases.
Apache Spark is a distributed computing framework widely used for big data processing and analytics. Distributed means Spark applications can executed across 1 or multiple nodes. The pro of distribution is performance and scale while the con is that distributed can make things a bit more challenging for developers and operators.
With an efficient processing engine and APIs that have become easier to use over time, Spark enables developers to handle large-scale data processing tasks.
PySpark, the Python API for Apache Spark, provides Python developers with an interface to leverage the capabilities of Spark from Python.
In this tutorial, we will provide a quick start guide to PySpark, covering the essential concepts and demonstrating basic operations with sample code.
Table of Contents
- PySpark Overview
- Next Steps
- Spark Context and Spark Session
- Actions and Transformations
- PySpark Quick Start Conclusion
PySpark Overview
In this PySpark quick start, let’s run some examples to cover basic fundamentals. We are going to run a variety of examples to get you started.
Requirements
Before diving into PySpark, ensure you have the following prerequisites:
- Python installed on your machine (version 3.x recommended).
- Apache Spark downloaded and extracted – see https://spark.apache.org/downloads.html. In examples below, I downloaded and extracted Spark 3.4 pre-built for hadoop 3
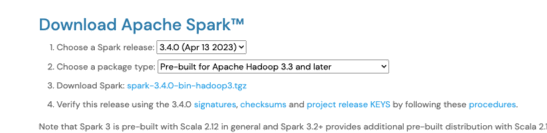
PySpark Quick Start Steps
Assuming you have downloaded and extracted Spark, we will use the PySpark shell to run PySpark code. On Mac or Linux, the PySpark shell is pyspark
located in the bin
directory. Starting the shell on my machine looks like this:
$ pwd
/Users/toddmcg/dev/spark-3.4.0-bin-hadoop3
$ bin/pyspark
Python 3.9.6 (default, Oct 18 2022, 12:41:40)
[Clang 14.0.0 (clang-1400.0.29.202)] on darwin
Type "help", "copyright", "credits" or "license" for more information.
Setting default log level to "WARN".
To adjust logging level use sc.setLogLevel(newLevel). For SparkR, use setLogLevel(newLevel).
23/05/24 06:38:14 WARN NativeCodeLoader: Unable to load native-hadoop library for your platform... using builtin-java classes where applicable
23/05/24 06:38:14 WARN Utils: Service 'SparkUI' could not bind on port 4040. Attempting port 4041.
Welcome to
____ __
/ __/__ ___ _____/ /__
_\ \/ _ \/ _ `/ __/ '_/
/__ / .__/\_,_/_/ /_/\_\ version 3.4.0
/_/
Using Python version 3.9.6 (default, Oct 18 2022 12:41:40)
Spark context Web UI available at http://192.168.1.16:4041
Spark context available as 'sc' (master = local[*], app id = local-1684928294920).
SparkSession available as 'spark'.
>>>
Creating a DataFrame
A DataFrame is a distributed collection of data organized into named columns, similar to a table in a database. DataFrame is the descendant of Resilient Distributed DataSet, which you will often see referenced. See Spark RDDs for more info. For now, let’s just continue with DataFrames as an abstraction to our underlying data.
PySpark provides APIs to create DataFrames from various sources, such as CSV files, JSON data, or existing RDDs. Let’s create a DataFrame from a list of dictionaries:
>>> data = [
... {"name": "Alice", "age": 25},
... {"name": "Bob", "age": 30},
... {"name": "Charlie", "age": 35}
... ]
>>> df = spark.createDataFrame(data)
For exploration of using Dataframes with specific sources in PySpark, see dedicated tutorials such as PySpark CSV, PySpark JSON, PySpark mySQL JDBC, etc.
Inspecting Data
To examine the contents of a DataFrame, you can use the show()
method, which displays a sample of the data:
>>> df.show()
+---+-------+
|age| name|
+---+-------+
| 25| Alice|
| 30| Bob|
| 35|Charlie|
+---+-------+
Performing Operations on DataFrames
PySpark provides operations to manipulate and transform DataFrames. Let’s explore a few common operations:
Selecting Columns
You can select specific columns from a DataFrame using the select()
method:
>>> # Select the "name" column from the DataFrame
>>> names = df.select("name")
>>>
>>> # Display the selected column
>>> names.show()
+-------+
| name|
+-------+
| Alice|
| Bob|
|Charlie|
+-------+
Filtering Rows
To filter rows based on a condition in PySpark, you can use the filter()
or where()
methods:
>>> # Filter rows where age is greater than 30
>>> filtered = df.filter(df.age > 30)
>>>
>>> # Display the filtered DataFrame
>>> filtered.show()
+---+-------+
|age| name|
+---+-------+
| 35|Charlie|
+---+-------+
Aggregating Data
PySpark supports various aggregation functions, such as count()
, sum()
, avg()
, etc.
>>> average_age = df.selectExpr("avg(age)").collect()
>>> print("Average age:", average_age)
Average age: [Row(avg(age)=30.0)]
>>> average_age = df.selectExpr("avg(age)").collect()[0][0]
>>> print("Average age:", average_age)
Average age: 30.0
Dataframe Joins in PySpark
You can perform joins on DataFrames using the join()
method. Let’s join two DataFrames based on a common column
>>> # Create another DataFrame with additional information
>>> data2 = [
... {"name": "Alice", "city": "New York"},
... {"name": "Bob", "city": "San Francisco"},
... {"name": "Charlie", "city": "London"}
... ]
>>> df2 = spark.createDataFrame(data2)
>>> # Join the two DataFrames on the "name" column
>>> joined = df.join(df2, "name")
>>>
>>> # Display the joined DataFrame
>>> joined.show()
+-------+---+-------------+
| name|age| city|
+-------+---+-------------+
| Alice| 25| New York|
| Bob| 30|San Francisco|
|Charlie| 35| London|
+-------+---+-------------+
For a deeper dive on joining in PySpark, see PySpark Joins with SQL or PySpark Joins with Dataframes.
Next Steps
The remainder of this post will cover Spark Core concepts. Spark Core is what makes all other aspects of the Spark ecosystem possible including Spark SQL, Spark Streaming, MLLib.
Spark Context and Spark Session
Most recently, the way to interact with Spark is via a SparkSession. In the early days it use to be SparkContext, so similar to DataFrames and RDDs, you will likely see references to both.
The examples above used the PySpark shell which provides both a SparkContext and SparkSession automatically. When you start pyspark, do you notice the last two lines:
Spark context available as 'sc' (master = local[*], app id = local-1684928294920). SparkSession available as 'spark'.
That’s how we’re able to use spark
from the examples.
When I originally wrote this tutorial, I wrote
“After obtaining a SparkContext, developers interact with Spark’s primary data abstraction called Resilient Distributed Datasets.
Resilient Distributed Datasets (RDDs) are an immutable, distributed collection of elements. These collections may be parallelized across a cluster. As we witnessed, RDDs are loaded from an external data set or created via a SparkContext.”
So, that’s old now. Now, as shown above, the primary data abstraction is a DataFrame and developers should use a SparkSession.
I know this can be confusing if you are starting PySpark and don’t know the history yet. See SparkContext vs. SparkSession vs. SQLContext for further explanation.
Actions and Transformations
When working with a Spark DataFrames (or RDDs), there are two available operations: actions or transformations.
An action is an execution which produces a result. Examples of actions in previous are count, first.
Example Spark Actions in Python
ut.count() // number of lines in the CSV file
ut.first() // first line of CSV
See PySpark Actions tutorial for deeper dive.
Example Spark Transformations in Python
Transformations create new DataFrames (RDDs) using existing Dataframes (RDDs). See PySpark transformations tutorial for further exploration.
PySpark Quick Start Conclusion
In this tutorial, we provided a quick start guide to PySpark, introducing Python developers to the powerful world of Apache Spark. We covered essential concepts, such as creating a SparkSession, creating DataFrames, performing operations on DataFrames, and joining DataFrames. With this foundation, you can explore more advanced features and unleash the full potential of PySpark for big data processing and analytics.
Full list of PySpark Tutorials
Looking for something in particular? Let me know in the comments below or feel free to reach out directly.