In this pyspark read csv tutorial, we will use Spark SQL with a CSV input data source using the Python API. We will continue to use the Uber CSV source file as used in the Getting Started with Spark and Python tutorial presented earlier.
Also, this Spark SQL CSV tutorial assumes you are familiar with using SQL against relational databases directly or from Python. So, in other words, you have experience with SQL and would like to know how to use with Spark.
Why Read CSV files in PySpark?
Reading CSV files in PySpark can be beneficial for several reasons:
- Scalability: PySpark is designed to handle large datasets and can distribute the processing of data across multiple nodes in a cluster. This makes it an ideal tool for processing large and/or many CSV files.
- Data manipulation: PySpark provides a powerful API for manipulating data, allowing you to perform complex transformations on CSV data. This can be useful for tasks such as data cleaning, data transformation, and data aggregation as we will see in tutorial below.
- Integration: PySpark can easily integrate with other big data tools and frameworks. This makes it a versatile tool for processing CSV data in a big data environment.
Reading CSV files in PySpark can be a powerful tool for processing large datasets and performing complex data transformations. Let’s find out.
PySpark Overview
Spark SQL uses a type of Resilient Distributed Dataset called DataFrames. DataFrames are composed of Row objects accompanied by a schema which describes the data types of each column. A DataFrame may be considered similar to a table in a traditional relational database. As you might expect, DataFrames may be created from a variety of input sources including CSV text files.
This intro to PySpark SQL post will use a CSV file from previous Spark Python tutorials found here:
PySpark Read CSV Depends on Spark Version
Your PySpark read csv approach depends on the version of Spark being used.
If you are an old version of Spark ( < Spark 2.0) the spark-csv package available from Spark Packages was released to make your lives easier, but it’s not a requirement to show pyspark reading csv. The spark-csv package is described as a “library for parsing and querying CSV data with Apache Spark, for Spark SQL and DataFrames” This library is compatible with Spark 1.3 and above.
However, if you are using Spark 2.0 and above, the spark-csv package has now been included in as described in the spark-csv github repo.
Table of Contents
- Why Read CSV files in PySpark?
- PySpark Overview
- PySpark Read CSV Depends on Spark Version
- PySpark SQL CSV Examples Setup
- PySpark Read CSV with SQL Examples
- PySpark SQL with CSV More Advanced
- PySpark SQL Resource
- What are PySpark Read CSV alternatives?
- Further PySpark References
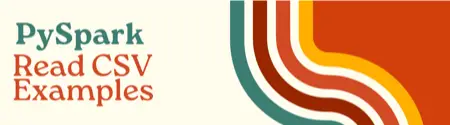
PySpark SQL CSV Examples Setup
To make things easier, we are going to dive the PySpark SQL examples with CSV files into two versions: either version of Spark less than 2.0 and another version of Spark 2.0 or above.
PySpark SQL CSV Example Setup < Spark 2.0
1. Depending on your version of Scala, start the pyspark shell with a packages command line argument.
At time of this writing, scala 2.10 version:
$SPARK_HOME/bin/pyspark --packages com.databricks:spark-csv_2.10:1.3.0
At time of this writing, scala 2.11 version:
$SPARK_HOME/bin/pyspark --packages com.databricks:spark-csv_2.11:1.3.0
2. Using the available sqlContext from the shell load the CSV read, format, option and load functions
>>> df = sqlContext.read.format('com.databricks.spark.csv')
.options(header='true', inferschema='true')
.load('Uber-Jan-Feb-FOIL.csv')
In the above code, we are specifying the desire to use com.databricks.spark.csv format from the package we passed to the shell in step 1. “header” set to true signifies the first row has column names. “inferSchema” instructs Spark to attempt to infer the schema of the CSV and finally load function passes in the path and name of the CSV source file. In this example, we can tell the Uber-Jan-Feb-FOIL.csv file is in the same directory as where pyspark was launched.
Note: you may be interested in comparing this example above to the Spark >= 2.0 version below. There is a csv
function now which makes things more convenient.
3. Register a temp table
>>> df.registerTempTable("uber")
Similar to how the spark-csv package requirement has changed over time, the registerTempTable
function has also changed. It is no longer used in Spark 2.0 and above as shown in the next setup example.
PySpark SQL CSV Example Setup > Spark 2.0
1. Start PySpark
~/dev/tmp $pyspark
Python 3.9.12 (main, Mar 26 2022, 15:51:15)
[Clang 13.1.6 (clang-1316.0.21.2)] on darwin
Welcome to
____ __
/ __/__ ___ _____/ /__
_\ \/ _ \/ _ `/ __/ '_/
/__ / .__/\_,_/_/ /_/\_\ version 3.3.1
/_/
Using Python version 3.9.12 (main, Mar 26 2022 15:51:15)
Spark context Web UI available at http://192.168.1.15:4040
Spark context available as 'sc' (master = local[*], app id = local-1669304534671).
SparkSession available as 'spark'.
>>>
This is just to show the Python and Spark version used in this setup.
2. Next, using the available SparkContext from the shell load the CSV read, format, options and csv functions
>>> df = spark.read.options(header='true', inferschema='true').csv('Uber-Jan-Feb-FOIL.csv')
In the options
function code above, the header
option being set to true signifies the first row has column names. Also, with inferSchema
being set to true, this instructs Spark to attempt to infer the schema of the CSV. Last, we use the csv
function to pass in the path and name of the CSV source file. In this example, we can tell the Uber-Jan-Feb-FOIL.csv file is in the same directory as where pyspark was launched.
3. Register a temp table
>>> df.createOrReplaceTempView("uber")
The createOrReplaceTempView
either creates or replaces a local, temporary view with the provided DataFrame. It used instead of registerTempTable
in newer versions of Spark. In either case, temporary views is similar in concept to SQL tables where each table contains rows and columns.
PySpark Read CSV with SQL Examples
We’re now ready to query using SQL such as finding the distinct NYC Uber bases in the CSV
>>> distinct_bases = sqlContext.sql("select distinct dispatching_base_number from uber")
>>> for b in distinct_bases.collect(): print b
Row(dispatching_base_number=u'B02598')
Row(dispatching_base_number=u'B02764')
Row(dispatching_base_number=u'B02765')
Row(dispatching_base_number=u'B02617')
Row(dispatching_base_number=u'B02682')
Row(dispatching_base_number=u'B02512')
5. It might be handy to know the schema
>>> df.printSchema()
root
|-- dispatching_base_number: string (nullable = true)
|-- date: string (nullable = true)
|-- active_vehicles: integer (nullable = true)
|-- trips: integer (nullable = true)
PySpark SQL with CSV More Advanced
Let’s try some more advanced SQL, such as determining which Uber bases is the busiest based on the number of trips
>>> sqlContext.sql("""select distinct(`dispatching_base_number`),
sum(`trips`) as cnt from uber
group by `dispatching_base_number`
order by cnt desc""").show()
+-----------------------+-------+
|dispatching_base_number| cnt|
+-----------------------+-------+
| B02764|1914449|
| B02617| 725025|
| B02682| 662509|
| B02598| 540791|
| B02765| 193670|
| B02512| 93786|
+-----------------------+-------+
Or the 5 busiest days based on the number of trips in the time range of the data:
>>> sqlContext.sql("""select distinct(date),sum(trips) as cnt
from uber group by date
order by cnt desc limit 5""").show()
+---------+------+
| date| cnt|
+---------+------+
|2/20/2015|100915|
|2/14/2015|100345|
|2/21/2015| 98380|
|2/13/2015| 98024|
|1/31/2015| 92257|
+---------+------+
PySpark SQL Resource
An older version of ipython notebook file https://github.com/tmcgrath/spark-with-python-course/blob/master/Spark-SQL-CSV-with-Python.ipynb
What are PySpark Read CSV alternatives?
There are several alternatives to reading CSV files in PySpark, depending on your specific use case and requirements:
- Pandas: Pandas is a popular Python library for data manipulation and analysis, which includes a
read_csv()
method for reading CSV files. Pandas can be used to process smaller CSV files that can fit into memory, and provides an API for data manipulation and analysis. - Dask: Dask is a distributed computing library for Python providing a Pandas-like API for parallel processing of large datasets. Dask can be used to read and process CSV files that are too large to fit into memory, and can scale to handle datasets that are larger than the available memory.
- Apache Flink: Apache Flink is a distributed computing framework for processing large-scale data streams and batch data processing. Flink can be used to read and process CSV files in a distributed environment, and provides a powerful API for data manipulation and analysis.
- Apache NiFi: Apache NiFi is a data integration and processing tool that supports the ingestion, processing, and delivery of data from various sources. NiFi includes processors for reading and processing CSV files, and can be used to integrate CSV data with other data sources and systems.
Overall, the choice of alternative to reading CSV files in PySpark depends on the specific use case and requirements, and may involve a trade-off between performance, scalability, and ease of use.
Further PySpark References
Featured image based on https://flic.kr/p/4FbcGr
Are we able to read a csv file directly from HTTPS, instead of the need to download that csv file to pyspark directory?