Change Data Capture is a mechanism to capture the changes in databases so they may be processed someplace other than the database or application(s) which made the change.
This article will explain what change data capture (CDC) is, how it works, and why it’s important for businesses.
Why? Why would we want to capture changes in databases?
Let’s cover common questions and concerns around Change Data Capture in this post, so we have context for the specific CDC tutorials later.
Also, in addition to “Why CDC”, let’s provide insight into other common CDC questions such as “What is CDC?” and “How do we implement CDC?” and listing any pros/cons to CDC.
- Why Change Data Capture?
- What is Change Data Capture?
- Change Data Capture Vendor Examples
- When to Consider Change Data Capture?
- Change Data Capture Vendor Options
Why Change Data Capture?
Let’s consider “Why CDC” at a high level first before we get into more of the details.
If data can be processed, which results in “value”, without any integration with other data, there is simply no need for CDC. Let’s consider “value” to be examples such as providing the ability better decisions, create more sales, improve logistic costs, etc. If we can realize this value in one application with one database, for example, there is no need for CDC.
What do we do if this “value” we are seeking can only be realized if data is combined from multiple sources? For example, how do we integrate data from an E-commerce application and our inventory databases? As a Data Engineer, how do you implement this? As you already know, you have many options such as traditional Extract Transform Load (ETL) or more modern Extract Load Transform (ELT) attempts.
What is Change Data Capture?
Change Data Capture extracts changes in online transactional processing (OLTP) data systems to downstream systems such as an Event Log, data lakes, and/or stream processors. For example, our CDC architecture might resemble this diagram.
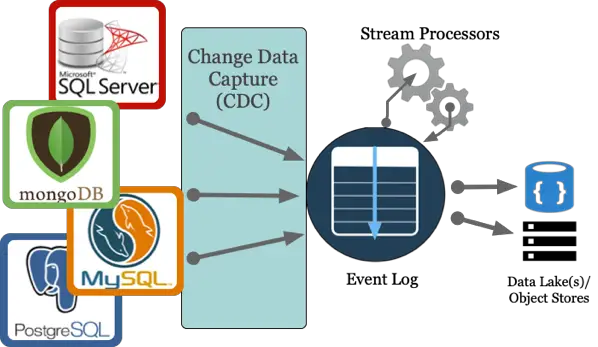
In essence, CDC is implemented in databases by writing to immutable transaction logs and then providing a mechanism to read from these logs. These transaction logs are not designed for CDC, but more often, these transaction logs are an unexpected benefit for CDC. These transaction logs are intended to solve resiliency and performance concerns in a particular database.
These logs also provide a mechanism for which a CDC process can read transactions with minimal performance impact on the transaction database.
- Turning on and configuring CDC also requires no change to existing schema or applications using the database.
- In addition to data mutations, CDC transaction logs can also capture changes in structure. In other words, a database transaction logs can capture both DML and DDL.
The alternative to CDC is reading from a transaction log is reading the database tables directly. The tables will need indicators to help determine what data has been created or updated. These indicators are typically implemented with audit columns such as dateCreated
to indicate Inserts and lastUpdated
to flag updates.
Let’s consider a high-level example. In an E-commerce application, new inserts, updates, or deletes to transactions in the E-commerce database could be consumed via CDC and sent to an Event Log. From here, the Event Log could be a source for Stream Processors to perform valuable near real-time computations such as fraud detection, recommendations, alerts, etc. or the Event Log could be just a plain old data buffer before publishing to downstream analytic systems.
For more information on Event Log, see Why Event Logs? post.
Change Data Capture Vendor Examples
Change Data Capture is freely available out-of-the-box from database vendors such as Microsoft SQL Server, Oracle, PostgreSQL, and MySQL.
In Microsoft SQL Server, CDC records insert, edit, and delete activities in the SQL Server table in a detailed format [1]. Column information, along with the metadata, is captured for the modified rows. Then, it is stored in append-only change tables. Table-valued functions enable users to have systematic access to these change data tables. Records within the change tables are immutable and therefore similar to the value of an immutable log.
In Oracle, it is possible to capture and publish changed data in synchronous and asynchronous modes [2]. In synchronous mode, change data is captured as part of the transaction that modifies the source table. SYNC_SOURCE is a single, predefined synchronous change source that cannot be altered.
Synchronous mode is cost-efficient, though it adds overhead to the source database at capture time. In asynchronous mode, change data is captured from the database redo log files after changes have been made to the source database.
In MySQL, CDC is available as part of the `binlog` which was originally used in auditing and copying data to other MySQL systems [3]. But, the `binlog` may be utilized outside of MySQL for CDC events processing and saving to downstream analytics systems for example.
In PostgreSQL, change data capture is possible in either transaction logs or triggers. In transaction logs, all the write transactions (i.e., INSERT, UPDATE, DELETE, DDL’s) are written to the Write-Ahead Logs (WAL) before the transaction result is sent to the user or client [4]. The WAL allows the consumption of CDC events.
In MongoDB, the transaction log is called the `opLog`.
When to Consider Change Data Capture?
Change data capture plays a significant role in streaming data processing and pipelines. As the amount of data grows rapidly, the need for CDC techniques becomes crucial to handle data inflow for analytics and near real-time analytics such as machine learning and artificial intelligence (AI).
Problems arise when long-running, taxing analytic queries are conducted on OLTP systems which affect overall application performance. By using CDC-based technologies, it allows users to capture database mutations such as inserts, updates, deletes as well as changes in structures such as DDL `alter table` changes automatically. This promotes flexibility to process in streaming applications and/or to store in destinations suited for analytic queries.
Advantages of Change data capture:
- CDC implementation does not require application code changes
- Only requires configuration changes to the database
- Enables us to identify a change history
- Facilitates the user to add context information to every DML data mutation if required
- CDC has an auto cleanup feature, deleting information automatically based on the retention period
Disadvantages of Change Data Capture:
- CDC may not track change time
- May not track the security context of the change
- Does not specify save how the data changed; it only tracks that a change was made
- A slight overhead to the system may be added, depending on the number of changes
Change Data Capture Vendor Options
Once you have configured CDC in Oracle, SQL Server, etc. you may be wondering how can you consume and process the CDC events? How can you implement a streaming architecture with CDC? What are your options for building CDC stream processors? etc.
At the time of this writing, here are a few options to consider and not listed in any particular order
- Debezium – Open Source. Built on the Kafka Connect framework. All of Debezium’s connectors are Kafka Connector source connectors so they have the pros and cons associated with Kafka Connect.
- StreamSets – Open Source. Out of the box support for all CDC implementations described here as well as others.
- Others? Let us know.
Also, you may consider vendor-specific options as well such as
- Oracle Golden Gate
- Dynamo DB Streams
- Attunity
Conclusion
Change data capture is available out-of-the-box in many database systems such as MS SQL Server, Oracle, MySQL, Postgres, and MongoDB. CDC is prevalent in streaming architectures when implementing separation of concerns between transactions and other concerns such as analytics, search indexing, machine learning, AI, near real-time monitoring, and alerting.
Hope this helps!
Let us know if you have any questions or concerns.
References
[1] SQL Server https://docs.microsoft.com/en-us/sql/relational-databases/track-changes/about-change-data-capture-sql-server?view=sql-server-2017
[2] Oracle CDC https://docs.oracle.com/cd/E11882_01/server.112/e25554/cdc.htm
[3] MySQL binlog https://dev.mysql.com/doc/internals/en/binary-log-overview.html
[4] PostgreSQL WAL https://www.postgresql.org/docs/11/runtime-config-wal.html
[6] Open Source Based Change Data Capture
Image credit https://pixabay.com/en/deer-dream-animal-fantasy-1333814/